DeepVOX: Discovering Features from Raw Audio for Speaker Recognition in Non-ideal Audio Signals
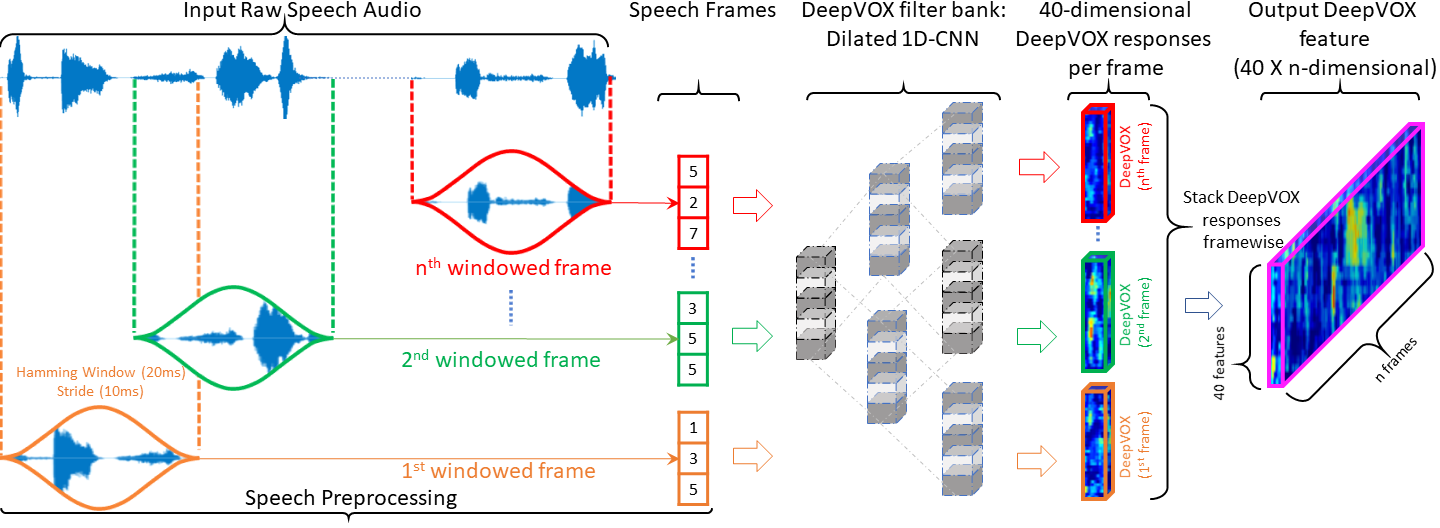
Automatic speaker recognition algorithms typically use pre-defined filterbanks, such as Mel-Frequency and Gammatone filterbanks, for characterizing speech audio. However, it has been observed that the features extracted using these filterbanks are not resilient to various types of audio degradations. In this work, we propose a deep learning-based technique, called DeepVOX, to deduce the filterbank design from vast amounts of speech audio. The purpose of such a filterbank is to extract features robust to non-ideal audio conditions, such as degraded, short duration, and multi-lingual speech. Experimental results on a large variety of speech datasets demonstrate the efficacy of the DeepVOX features across various degraded, short-duration, and multi-lingual speech. Furthermore, the DeepVOX features also improve the performance of existing speaker recognition algorithms, such as the xVector-PLDA and the iVector-PLDA.